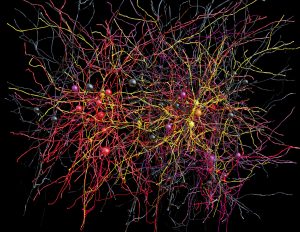
Artificial intelligence has progressed immensely in the past decade with the fantastic open source nature of the community. However there are relatively few people, even in the research areas, that understand the history of the field from both the computational and biological standpoints. Standing on the shoulders of giants is a great way to step forward, but can you truly innovate without understanding the fundamentals?
I go to a lot of conferences and I’ve noticed a subtle change in the past few years. Solutions that are being spoken about now don’t appear to be as far forward as some of those presented a couple of years ago. This may be subjective, but the more I speak to people about my own background in biochemically accurate computational neuron models, the more interest it sparks. Our current deep learning model neurons are barely scratching the surface of what biological neurons can do. Is it any wonder that models need complexity and are limited in their scope?
In June, I gave a talk on this exact topic (Creating AI using Biological Network Techniques ) at Ravensbourne as part of London Tech Week: looking at the complexity of the biological synapse and the multiple dimensions of control, compared to the single connection weights that exist in the current TensorFlow models. It’s been a while since I gave such an abstract talk1 and I really enjoyed it2. From the feedback I had on the night, it was clear that these ideas were new to a lot of people. My PhD was looking into creating network models in the computer that behaved exactly the same as physical neurons when stimulated by current or (simulated) chemical washes. I used machine learning3 to find appropriate missing parameters. The whole project was a very enjoyable few years at the intersection of mathematics, biology and computing. What has surprised me since is how many PhD students and AI researchers don’t understand the original biology that inspired the AI giants (Benigo, Hinton, Ng and others) of today and I think this is why we haven’t seen any major leaps forward in results. It’s been evolution rather than innovation.
Demis Hassabis and team from DeepMind recently authored a review in Neuron on this exact topic. The full text is available4 here and it’s very well worth a read. Demis also earns some bonus points from me for having cited relevant papers from each of the past nine decades5 which really underlines how un-new this field is6. This also gives a hint to the community about how DeepMind are pushing forward – they are looking beyond what is in front of them and being inspired from nature. After all, what could beat a system that has had over a million epochs with an exponential increase in parallel runs currently at 7 billion…7.
“Human subtlety will never devise an invention more beautiful, more simple or more direct than does nature” Leonardo do Vinci
There are far too many papers published daily to keep on top of all the research in all the fields that might be relevant, but the more widely read you are and the more you discuss and debate scientific ideas with people from different backgrounds, the more likely you are to find that next big thing. You don’t have to be a polymath to work in artificial intelligence, but it helps!
- More recently I’ve spoken on Women in Tech and specific applications of technology. ↩
- I saw a lot of people taking pictures – if you have any please get in touch as no official photos were released. ↩
- Yes, even back then! ↩
- I firmly believe that full text of all scientific articles should be available. ↩
- I’m sure he could have found something from the late 1920s to get the full 10, but Turing’s 1936 paper is arguably the start. ↩
- There’s a whole extra post on my experiences in the world of scientific funding and how “trendy” subjects get 5-year grants easier than work that is no less critical, but less in the public eye. I hope that this has changed some what since I left academia. ↩
- Very approximately, assuming 2.5 million years back to genus homo which is ~83000 generations and guessing back further, although anything further back than early mammals ~ 300 million years ago is a non-functional code base in my opinion 😉 ↩
One of my favourite research projects was by Tom Ray called Tierra (https://en.wikipedia.org/wiki/Tierra_(computer_simulation)). It’s purpose was to evolve computer programs, and it had some interesting results.
The problem with using more neural inspired models is getting a suitable learning algorithm. Systems like Tierra used the genetic algorithm approach, but obviously that is orders of magnitude slower than back propagation.
I have a project on the back burner, which re-implements Tierra (or something similar to it) on a GPU to get the speed advantage of the massive parallelism that you can get even on consumer hardware.
Sounds like a great project! If there weren’t problems with this approach then the innovation would have already happened. One of the big advantages there is now over my own PhD is the use of GPUs – I think we have the hardware now to do some really exciting stuff. DeepMind are already pushing in this direction and I’d love to see some parallel innovation on this.